The Transformative Role of AI in Drug Discovery and Development: A Cytocast Perspective
2023-12-05 by Kinga Sükösd
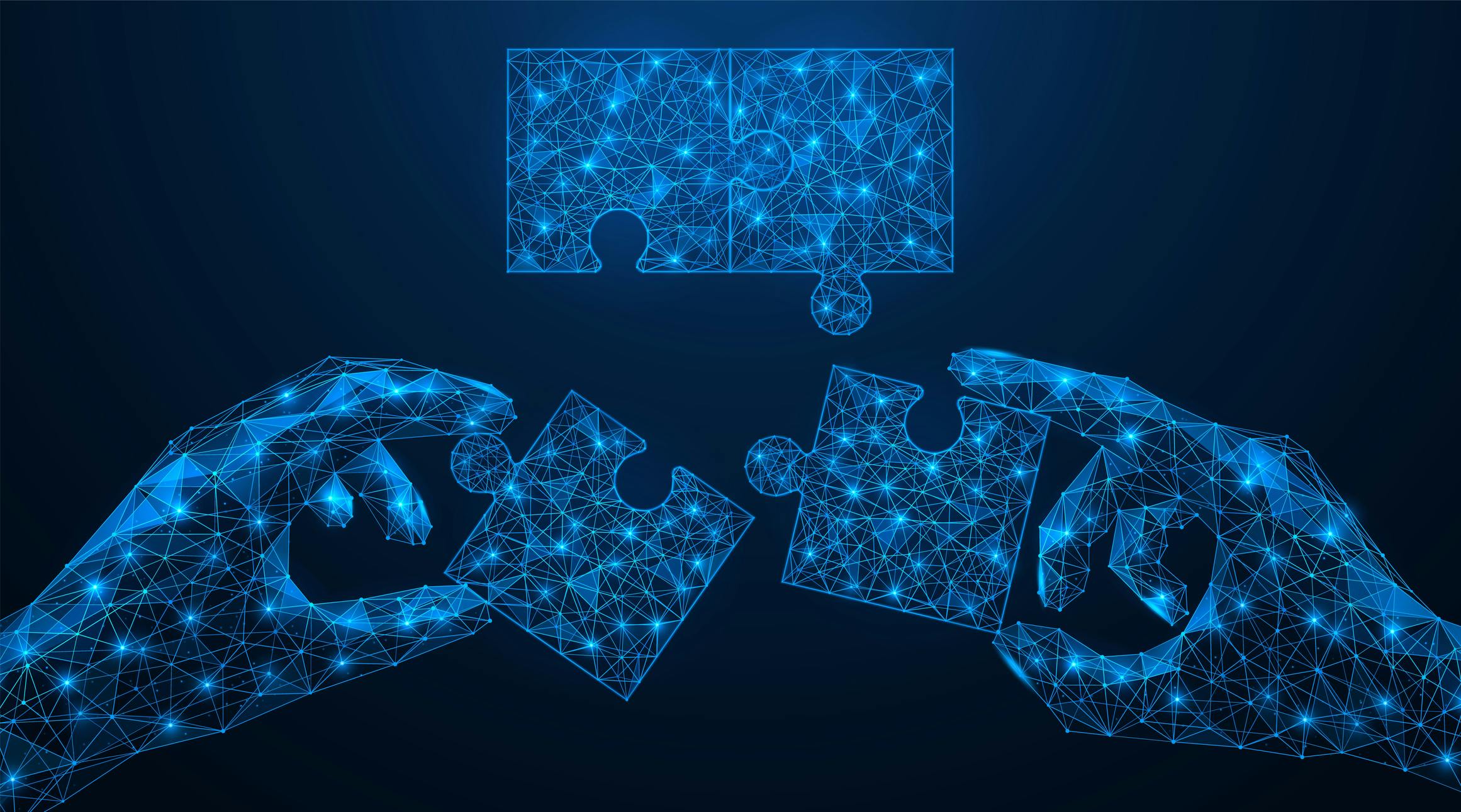
At Cytocast, integrating artificial intelligence (AI) methods propels our mission to accelerate the discovery and development of life-saving medications. Given the fast-evolving nature of this field, we aim to provide succinct yet informative perspectives. This includes covering fundamental methodologies that have influenced the domain, the primary challenges, and the promising opportunities it provides. Throughout this discussion, we'll integrate insights from our colleagues Santiago Noto and János Szalma, who play pivotal roles in the AI advancements for our product, the Cytocast Simulated Cell.
The human body is an incredibly complex system. Discovering just one new medicine to treat an illness successfully takes many years and it is a highly expensive process. Can AI change this? Absolutely!
Even before the emergence of generative AI models like ChatGPT, AI had already showcased its capability to revolutionize various phases of drug discovery, enhancing efficiency, accuracy, and speed. Its impact spans from drug design to market introduction, with AI models intricately mapping paths that integrate biology, chemistry, computational science, pharmacology, and disease treatments.
The evolution of AI in drug discovery and development has seen a progression from early chemoinformatics to the sophisticated applications of deep learning and generative models. Current trends shaping the future of AI highlight the integration of diverse data sources and a significant emphasis on interpretability. An illustrative example of this progress is evident in the creation of the first entirely AI-based preclinical drug candidate, INS018_055, designed to help treat idiopathic pulmonary fibrosis.
KEY METHODS
- Chemoinformatics and QSAR (Quantitative Structure-Activity Relationship)
In the early stages, chemoinformatics and QSAR played a crucial role. These methodologies focused on analyzing chemical structures and their activities, paving the way for computational predictions of drug-relevant properties.
- Machine Learning Approaches
As computational power increased, machine learning techniques gained prominence. Traditional algorithms, such as decision trees and support vector machines, were applied to analyze biological and chemical data, enabling predictive modeling and pattern recognition.
- Deep Learning and Neural Networks
The advent of deep learning marked a significant shift. Neural networks, particularly deep neural networks, demonstrated enhanced capabilities in learning complex representations from vast datasets. This allowed for more accurate predictions of drug-target interactions and bioactivity.
- Natural Language Processing (NLP)
NLP became increasingly important in extracting valuable information from scientific literature and textual data. This facilitated the identification of potential drug targets, pathways, and interactions, contributing to more informed decision-making.
- Generative Models and Reinforcement Learning
More recently, generative models and reinforcement learning have gained attention. These models, like generative adversarial networks (GANs), have shown promise in generating novel molecular structures with desired properties. Reinforcement learning has been employed to optimize drug candidates through iterative feedback processes.
- Integration of Multi-Omics Data
The integration of diverse omics data, including genomics, proteomics, and metabolomics, has become crucial. AI algorithms can analyze and interpret this wealth of information, aiding in a holistic understanding of disease mechanisms and drug responses.
- Explainable AI (XAI)
With the increasing complexity of AI models, the need for interpretability has grown. Explainable AI techniques aim to provide insights into the decision-making process of AI models, ensuring transparency and facilitating trust in the generated results.
CHALLENGES
AI-based drug discovery and development present several challenges that researchers and industry professionals must navigate. The successful application of AI hinges on factors like the availability of high-quality data, addressing ethical concerns, and recognizing the limitations inherent in AI-based approaches.
- Data Quality and Availability
One primary concern is the quality and diversity of the data used to train AI models. Biased or incomplete datasets can lead to skewed predictions, potentially jeopardizing the success of drug development programs.
- Interpretable AI Models
The interpretability of AI-generated insights remains a challenge, as understanding the rationale behind AI-driven predictions is crucial not just for gaining regulatory approval, but for decision-making processes and ensuring reliability and trustworthiness. These can be addressed through model interpretation and quantifying model uncertainty. Explainable AI is crucial for understanding what an AI model has learned and why it makes specific predictions, especially in the intricate field of biology. Additionally, quantifying model uncertainty allows researchers to gauge when the model is making informed predictions and when it is navigating uncharted territory.
- Ethical and Regulatory Concerns
Another challenge lies in the ethical considerations surrounding AI in drug development. Issues related to data privacy, biases, informed consent, and the responsible use of AI technologies must be carefully addressed to ensure the ethical conduct of research and development processes.
- Integration of Multi-Omics Data
Integrating diverse omics data, such as genomics, proteomics, and metabolomics, requires sophisticated AI approaches. A coherent analysis of these varied data types is essential for a comprehensive understanding of disease mechanisms.
- Cost and Resource Intensiveness
We cannot forget that AI-based solutions can be resource-intensive in terms of computing power, skilled personnel, and financial investment.
- Validation and Reproducibility
Ensuring the reproducibility and validation of AI models in different settings is essential for their successful application in drug discovery. When the emphasis is not solely on generating novel molecules; ensuring their safety is paramount. Validation of generated molecules is crucial, with AI playing a role in predicting toxicity before experimental validation.
FUTURE PERSPECTIVES
The future of AI in drug discovery holds immense promise. Advanced AI models, including deep learning architectures, are expected to further enhance the accuracy and efficiency of predictive models. The integration of AI with other cutting-edge technologies may open new frontiers in drug discovery by solving complex computational problems at unprecedented speeds. The ultimate goal is to leverage generative AI to design therapies tailored to individual patients.
- The utilization of AI in target identification and drug repurposing
By employing machine learning algorithms, researchers can analyze vast datasets to identify potential drug targets and repurpose existing drugs for new therapeutic indications. The ability to sift through massive amounts of biological and chemical data has streamlined the early stages of drug discovery, significantly reducing the time and resources required for target identification. The goal is ambitious yet promising: to generate valid molecules with potential therapeutic applications. - The application of AI in predictive toxicology
Traditional methods for assessing drug toxicity can be time-consuming and costly. AI, however, enables the prediction of potential toxicities through the analysis of diverse biological data, including genomics and proteomics. This not only expedites the drug development process but also enhances the safety profile of potential candidates.
- AI in drug formulation and optimization
AI algorithms can analyze complex interactions between drugs and biological systems, facilitating the design of more effective drug formulations. This precision in formulation enhances the therapeutic efficacy of drugs while minimizing side effects, a critical aspect in the development of novel pharmaceuticals. A particularly exciting direction is de novo drug design, where generative models could pave the way for the development of novel, synthetic molecules.
AI DEVELOPMENT AT CYTOCAST
Looking forward, the future of AI in drug discovery is dazzling. Enhanced predictive capabilities, refined personalized medicine approaches, and more efficient drug identification processes are on the horizon. Cytocast actively contributes to shaping this future by pioneering the use of AI to model drug-target interactions, predict drug efficacy, and identify potential side effects early in the drug developmental trajectory.
The Cytocast Simulated Cell integrates various AI methodologies discussed in the article. It harnesses the power of machine learning for predictive modeling and pattern recognition in biological data. Deep learning and neural networks play a pivotal role in accurately predicting drug-target interactions and bioactivity. Additionally, the integration of multi-omics data is a fundamental aspect, providing a holistic understanding of drug responses.
Staying ahead of the latest database releases is crucial, recognizing that the fuel for AI is high-quality, up-to-date data. Two key trends are shaping the AI landscape at Cytocast: the availability of more and better-quality data on increasingly smaller levels, such as the cellular level, and continuous breakthroughs in deep learning, including graph neural networks and large language models. The long-term vision is personalized medicine, aiming to tailor therapies to individual patients based on generative AI insights.
While addressing challenges such as data quality and interpretability, Cytocast upholds a commitment to ethical considerations and the responsible use of AI in drug development. Looking to the future, Cytocast envisions ongoing contributions to the field, with a specific focus on predictive toxicology and assessing drug effectiveness.
References:
- Qureshi, R., Irfan, M., Gondal, T. M., Khan, S., Wu, J., Hadi, M. U., ... & Alam, T. (2023). AI in Drug Discovery and its Clinical Relevance. Heliyon.
- Blanco-Gonzalez, A., Cabezon, A., Seco-Gonzalez, A., Conde-Torres, D., Antelo-Riveiro, P., & Pineiro, A. The Role of AI in Drug Discovery: Challenges, Opportunities, and Strategies. arXiv 2022.
- Zeng, X., Wang, F., Luo, Y., Kang, S. G., Tang, J., Lightstone, F. C., ... & Cheng, F. (2022). Deep generative molecular design reshapes drug discovery. Cell Reports Medicine.